Unsupervised Structure-Consistent Image-to-Image Translation, ISVC 2022
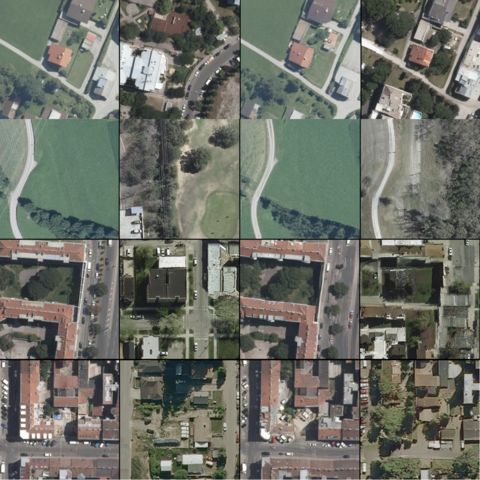
Our work on "Unsupervised Structure-Consistent Image-to-Image Translation
" has been accepted as a conference paper at the 17th International Symposium on Visual Computing (ISVC), 2022. The paper is co-authored by Shima Shahfar and Charalambos Poullis.
Abstract: The Swapping Autoencoder achieved state-of-the-art performance in deep image manipulation and image-to-image translation. We improve this work by introducing a simple yet effective auxiliary module based on gradient reversal layers. The auxiliary module's loss forces the generator to learn to reconstruct an image with an all-zero texture code, encouraging better disentanglement between the structure and texture information. The proposed attribute-based transfer method enables refined control in style transfer while preserving structural information without using a semantic mask. To manipulate an image, we encode both the geometry of the objects and the general style of the input images into two latent codes with an additional constraint that enforces structure consistency. Moreover, due to the auxiliary loss, training time is significantly reduced. The superiority of the proposed model is demonstrated in complex domains such as satellite images where state-of-the-art are known to fail. Lastly, we show that our model improves the quality metrics for a wide range of datasets while achieving comparable results with multi-modal image generation techniques.
Link to PDF: https://arxiv.org/abs/2208.11546