Transductive meta‑learning with enhanced feature ensemble for few‑shot semantic segmentation
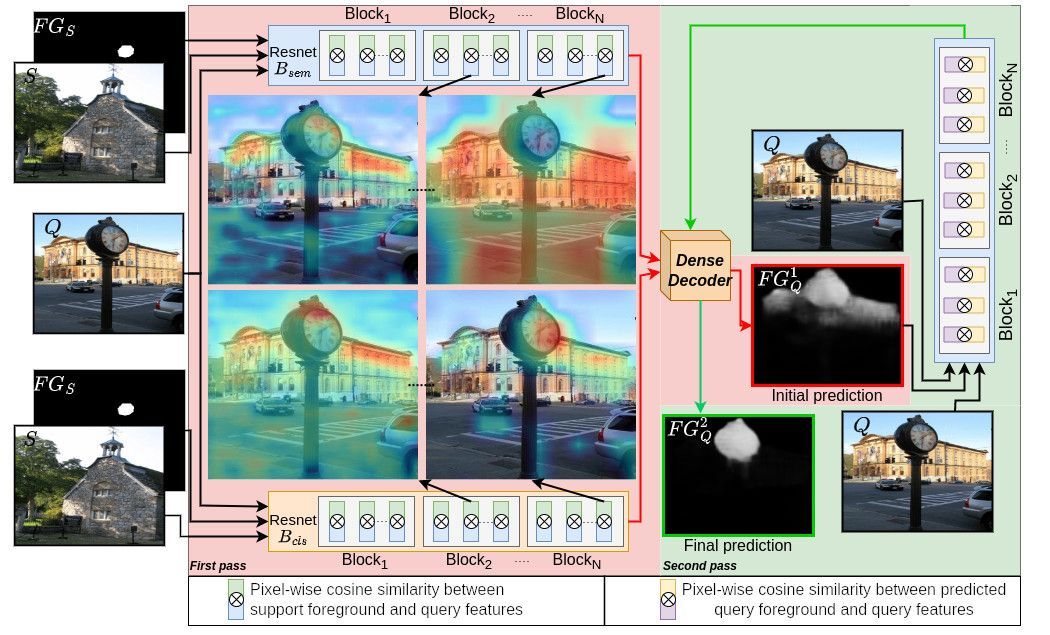
The paper "Transductive Meta-Learning with Enhanced Feature Ensemble for Few-shot Semantic Segmentation" by Amin Karimi and Charalambos Poullis has been accepted for publication in Scientific Reports.
TL;DR: We propose two-pass end-to-end method for few-shot semantic segmentation. The approach leverages an ensemble of visual features learned from pretrained classification Bcls and semantic segmentation Bsem networks with the same architecture. Bsem is also used as a base class extractor. The first pass matches support foreground features to query features to address intra-class variation, and the second pass suppresses false positives and propagates query foreground features to leverage intra-object variation.